Example: Reading 2D tabular data (DataFrame)#
This example illustrates the how to read 2D tabular data using the HydroMT DataCatalog with the csv
driver.
[1]:
# import hydromt and setup logging
from hydromt import DataCatalog
from hydromt.log import setuplog
logger = setuplog("tabular data example", log_level=10)
2024-06-14 08:21:19,323 - tabular data example - log - INFO - HydroMT version: 0.10.0
[2]:
data_catalog = DataCatalog("data/tabular_data_catalog.yml", logger=logger)
2024-06-14 08:21:19,328 - tabular data example - data_catalog - INFO - Parsing data catalog from data/tabular_data_catalog.yml
CSV driver#
time series data#
To read 2D tabular data from a comma-separated file (csv) and parse it into a pandas.DataFrame we use the pandas.read_csv(). Any driver_kwargs in the data catalog are passed to this method, e.g., parsing dates in the “time” colum and setting this as the index.
This works similarly for excel tables, but based on the pandas.read_excel() method.
For demonstration we use a dummy example timeseries data in csv.
[3]:
# inspect data source entry in data catalog yaml file
data_catalog["example_csv_data"]
[3]:
data_type: DataFrame
driver: csv
driver_kwargs:
index_col: time
parse_dates: true
path: /home/runner/work/hydromt/hydromt/docs/_examples/data/example_csv_data.csv
We can load any 2D tabular data using DataCatalog.get_dataframe(). Note that if we don’t provide any arguments it returns the full dataframe.
[4]:
df = data_catalog.get_dataframe("example_csv_data")
df.head()
2024-06-14 08:21:19,342 - tabular data example - dataframe - INFO - Reading example_csv_data csv data from /home/runner/work/hydromt/hydromt/docs/_examples/data/example_csv_data.csv
[4]:
col1 | col2 | |
---|---|---|
time | ||
2016-01-01 | 0.590860 | 0.591380 |
2016-01-02 | 0.565552 | 0.571342 |
2016-01-03 | 0.538679 | 0.549770 |
2016-01-04 | 0.511894 | 0.526932 |
2016-01-05 | 0.483989 | 0.502907 |
The data can be visualized with the .plot() pandas method.
[5]:
df.plot(y="col1")
[5]:
<Axes: xlabel='time'>
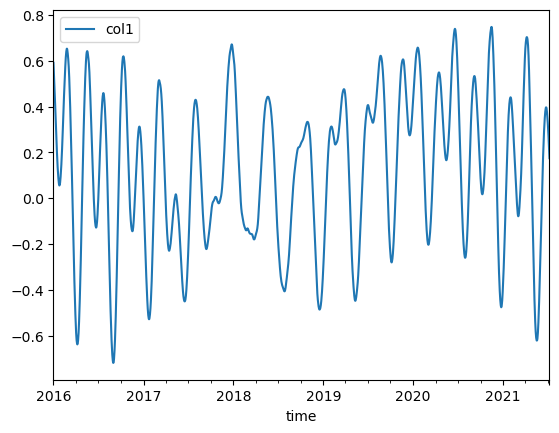
reclassification table#
Another typical usecase for tabular data are reclassification tables to reclassify e.g. land use data to manning roughness. An example of this data is shown in the cells below. Note tha the values are not validated and likely too high!
[6]:
# read both the vito_reclass and artifact_data data catalogs
data_catalog = DataCatalog(["data/vito_reclass.yml", "artifact_data"], logger=logger)
data_catalog["vito_reclass"]
2024-06-14 08:21:19,794 - tabular data example - data_catalog - INFO - Parsing data catalog from data/vito_reclass.yml
2024-06-14 08:21:19,835 - tabular data example - data_catalog - INFO - Reading data catalog artifact_data latest
2024-06-14 08:21:19,836 - tabular data example - data_catalog - INFO - Parsing data catalog from /home/runner/.hydromt_data/artifact_data/v0.0.9/data_catalog.yml
[6]:
data_type: DataFrame
driver: csv
driver_kwargs:
index_col: 0
meta:
notes: reclass table for manning values
path: /home/runner/work/hydromt/hydromt/docs/_examples/data/vito_reclass.csv
[7]:
df = data_catalog.get_dataframe("vito_reclass")
df.head()
2024-06-14 08:21:20,435 - tabular data example - dataframe - INFO - Reading vito_reclass csv data from /home/runner/work/hydromt/hydromt/docs/_examples/data/vito_reclass.csv
[7]:
description | landuse | manning | |
---|---|---|---|
vito | |||
0 | Unknown | 0 | -999.000 |
20 | Shrubs | 20 | 0.500 |
30 | Herbaceous vegetation | 30 | 0.150 |
40 | Cultivated and managed vegetation/agriculture ... | 40 | 0.200 |
50 | Urban / built up | 50 | 0.011 |
[8]:
da_lulc = data_catalog.get_rasterdataset("vito")
da_man = da_lulc.raster.reclassify(df[["manning"]])
da_man["manning"].plot.imshow()
2024-06-14 08:21:20,445 - tabular data example - rasterdataset - INFO - Reading vito raster data from /home/runner/.hydromt_data/artifact_data/v0.0.9/data.tar/vito.tif
[8]:
<matplotlib.image.AxesImage at 0x7f933ff0bd90>
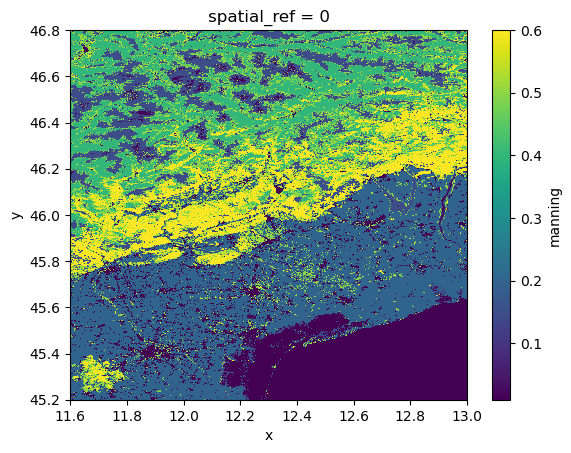